
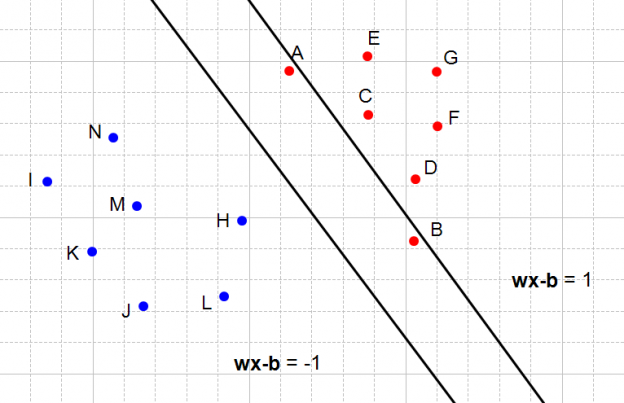
Sometimes, we might want to allow (on purpose) some margin of error (misclassification). A note about the Soft margin and C parameter If the decision boundary is too close to the support vectors then, it will be sensitive to noise and not generalize well. The decision boundary is drawn in a way that the distance to support vectors are maximized. In summary, SVMs pick the decision boundary that maximizes the distance to the support vectors. The best separating line is the yellow one that maximizes the margin (green distance). So, as stated before, for an 2-dimensional space the decision boundary is going to be just a line as shown below. In that space, the separating hyperplane is an (N-1)-dimensional subspace.Ī hyperplane is an ( N-1)- dimensional subspace for an N- dimensional space. To get the main idea think the following: Each observation (or sample/data-point) is plotted in an N-dimensional space with Nbeing the number of features/variables in our dataset. The SVCs aim to find the best hyperplane (also called decision boundary) that best separates (splits) a dataset into two classes/groups (binary classification problem).ĭepending of the number of the input features/variables, the decision boundary can be a line (if we had only 2 features) or a hyperplane if we have more than 2 features in our dataset. The decision boundary (separating hyperplane) In this article, I am not going to go through every step of the algorithm (due to the numerous amount of online resources) but instead, I am going to explain the most important concepts and terms around SVMs.
